THIS ARTICLE/PRESS RELEASE IS PAID FOR AND PRESENTED BY the University of Bergen - read more
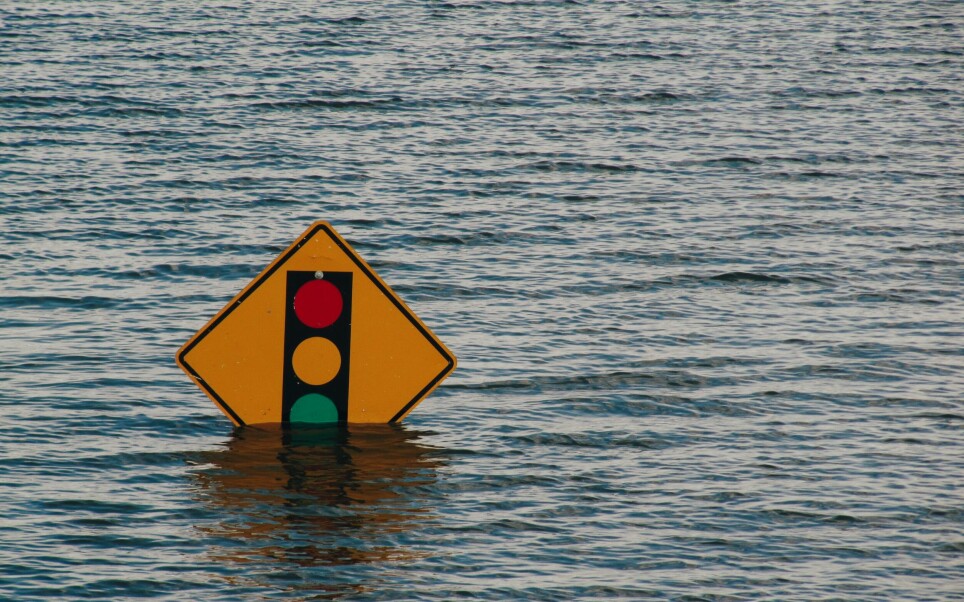
How can AI predict floods?
PODCAST: Norwegian researcher will take flood prediction to the next step by introducing artificial intelligence.
Throughout history, humans have always congregated around rivers, for the fresh water, food, and ease of transport they provide – so flooding has been an ever-present danger. As population increases, so does the exposure of people and property.
While good preparations can stave off the worst impacts of a flood, this requires knowledge of when a flood is coming. Emergent technologies like machine learning may hold the key to reliable flood prediction. Jenny Hagen studies this emerging technology, working with taking flood prediction the next step by introducing artificial intelligence.
“Machine learning is a sub-branch of artificial intelligence,” says Jenny Hagen, PhD and specialist on flood prediction with machine learning at the University of Bergen and the Bjerknes Centre.
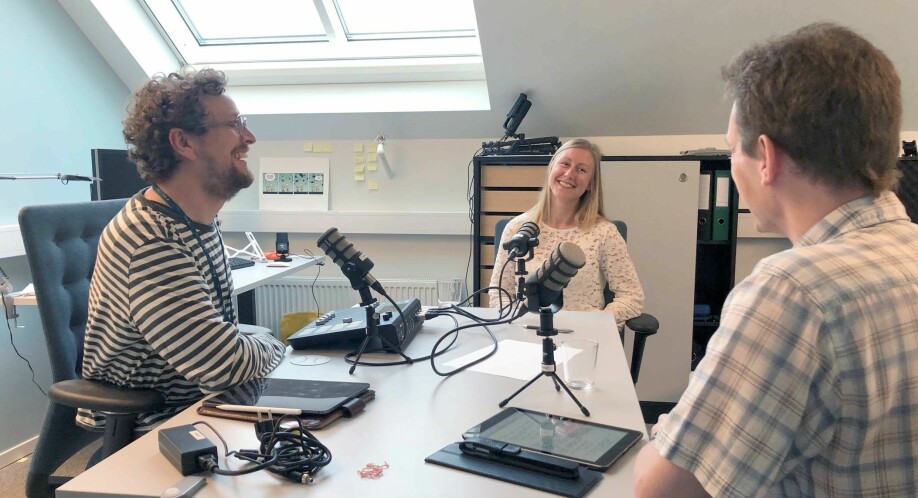
How is flood prediction done now?
In this podcast, she explains how machine learning can speed up and improve flood prediction, even in a changing climate, by letting the machine learn from historical data. But how is flood prediction done today?
“Flood prediction today, in the case of Norway, is handled by NVE in collaboration with the Meteorological Institute. Rainfall forecasts are fed to hydrological models that convert rainfall into discharge,” says Hagen.
Hydrological models are many and varied, but the simplest can be compared to a bucket. If the input is water, the output is whatever flows over the edges of the bucket – the discharge, or flow of water.
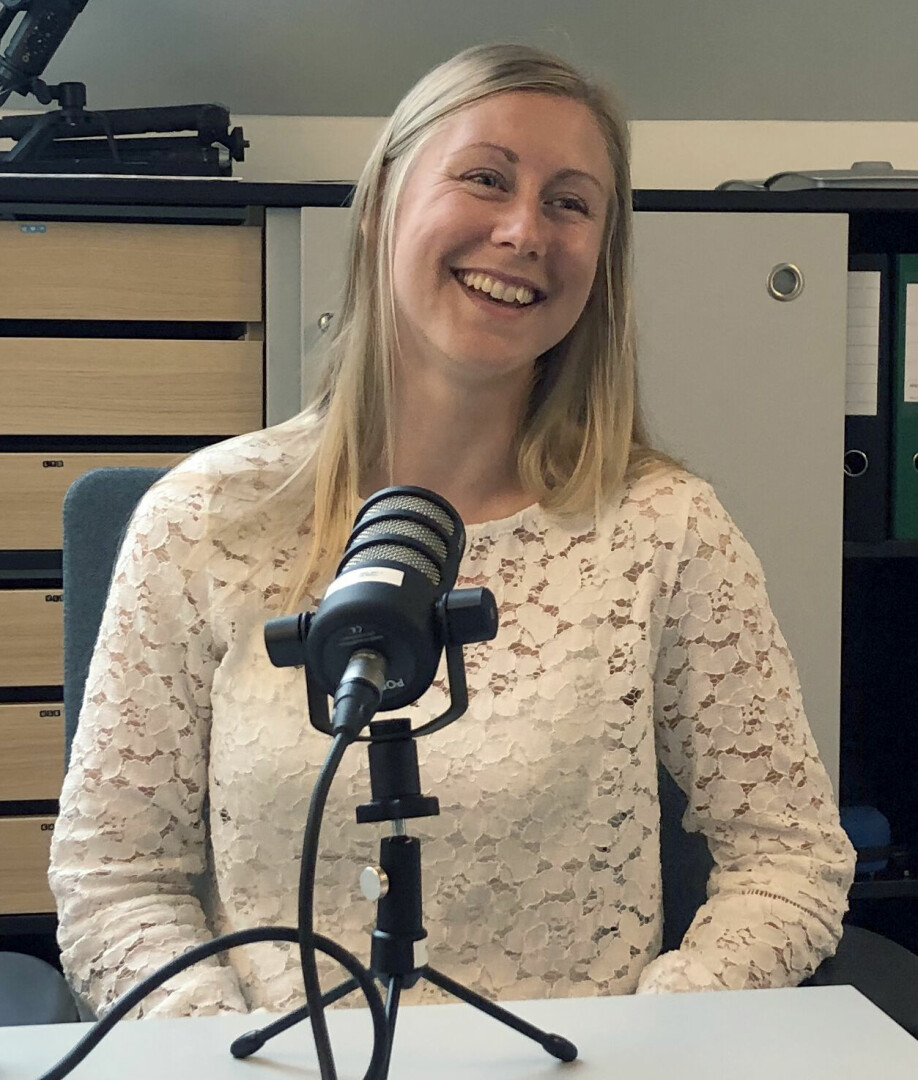
In reality, the models are more advanced, and take into account the slope, soil, and other properties of the landscape around the water.
“With this we can go further, and get the extent of flooding with the use of hydraulic models, which convert discharge into water coverage.”
In general, there is a consensus that wet areas will get wetter, and dry areas will get drier, in the future changing climate. For floods this means that they can intensify and become more frequent. But there are uncertainties, which needs a closer look.
How will machine learning change this?
One of the best things about machine learning is that these algorithms can go through tons of data that we humans could never have the time or resources to process.
Google Translate, new friend suggestions on Facebook, teaching robots movements by example – all are principles of machine learning in some way. Jenny's work focuses on the application of machine learning to link large-scale atmospheric circulation – pressure, rainfall, temperature, winds, and so on – to flooding in Norwegian catchments, as part of the Climate Hazards and Extremes project at the Bjerknes Centre.
“We use a simple analysis with three traditional machine learning techniques: random forest, support-vector machine and shallow neural networks,” says Hagen.
The three techniques are quite different in their structure, some are possible to take apart to see the inner workings, while others work as a black box – but all three models take the same kind of input – large-scale atmospheric variables – and produce the same kind of output – namely river discharge.
“The most important thing for a machine learning model is not the structure, but the data quality and quantity. If you put garbage in, of course you will get garbage out,” says Jenny.
The models are tested on real-life examples, hydrological stations in Norway, to see which perform best when predicting river streamflow.
The challenges for the future
Past studies with machine learning have looked at this on longer time scales, with monthly, seasonal, or yearly discharge. The new part in this work is looking at discharge prediction on the daily time scale.
“All the different rivers and catchments have different response times and linkages to large-scale atmospheric circulation. Some may be bigger and some may be smaller, some steeper and some mountainous, and some have snow as a driving factor of floods,” says Hagen, stating there is a clear difference in rainfall-driven and snowmelt-driven flood prediction.
“It is more difficult to accurately predict flooding in rainfall-driven flood regimes. This may have something to do with local variability and response times in relation to seasonal cycles."
For the future she is looking to use long-short term memory neural networks, expanding into deep learning:
“These actually have a sort of memory. The algorithm can remember sequences it has seen in the past, and utilise this to give better estimates of the river response to atmospheric forcing."
She sees this as the future of operational forecasts, stating that there are several initiatives that want to develop this further in Norway and Europe.
Listen to the podcast
The podcast is produced by Stephen Outten and Ingjald Pilskog. Stephen Outten is a researcher at Nansen Environmental and Remote Sensing Center and Bjerknes Centre for Climate Research. Ingjald Pilskog is an associated professor at Western Norway University of Applied Sciences and connected to the Bjerknes Centre for Climate Research.
Reference:
Jenny SjåstadHagen et.al.: Identifying major drivers of daily streamflow from large-scale atmospheric circulation with machine learning. Journal of Hydrology, 2021.
See more content from the University of Bergen:
-
Mini-brains may enable new treatments for brain disorders like epilepsy
-
Geese can impact the permafrost on Svalbard
-
Autumn arrives later underwater than before
-
Arctic lake archive: 10,000 years of storm data reveals a big surprise
-
This ice does not get the chance to grow old
-
Researchers play hide and seek with CO2