An article from Gjøvik University College
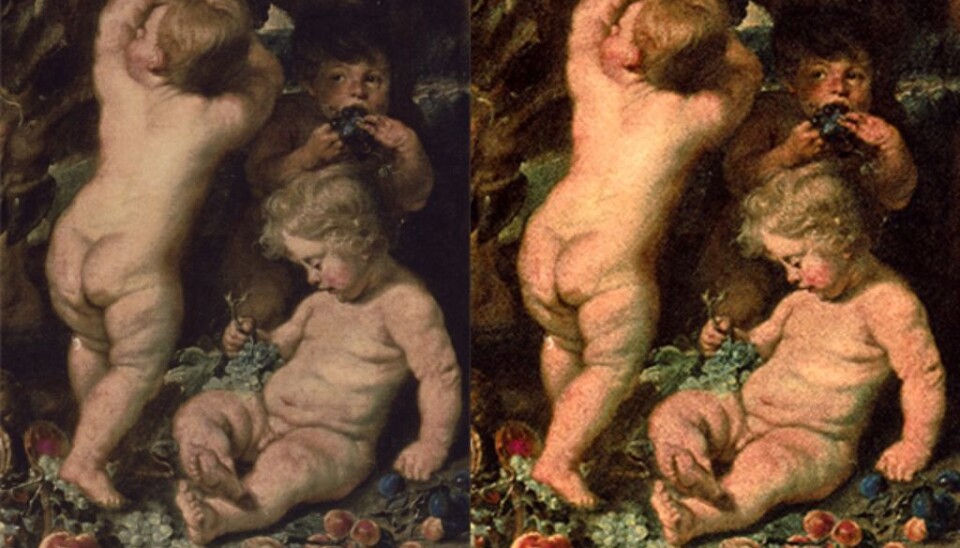
Photo imaging brings new life to missing artwork
Advanced mathematics and colour science can recreate artwork we thought was lost forever.
Denne artikkelen er over ti år gammel og kan inneholde utdatert informasjon.
Throughout history, many priceless works of art has been stolen or destroyed. No later than last summer it was announced that Monet's Waterloo Bridge, London and Picasso's Harlequin Head was destroyed in a fire in Romania.
Today, our main source of information and knowledge about many lost works of art, are photographs in older art books. Many of these photographs stems from the early 1900s. They are not considered particularly good, the picture quality is low, the colour rendering is incorrect and the resolution is low. Yet they are unique as the only source of many artworks that are missing.
Wouldn’t it be of great interest, not just for the art world, but also the ordinary art enthusiast, to have the possibility to admire a reproduction of the original piece of art, in high resolution and with as naturally rendered colours as possible? Researchers in this field do not have an easy starting point, but it is possible.
Better insight into lost works of art
There are many ways to preserve and recreate art.
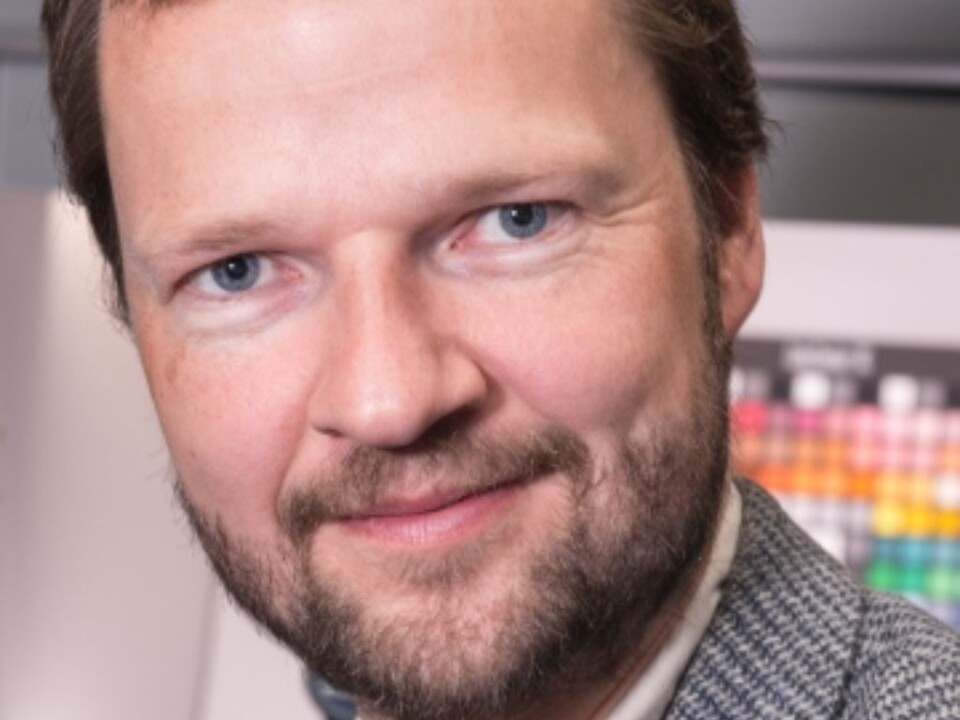
Researchers at Gjøvik University College (GUC) in Norway are developing a new method to recuperate high quality images of artworks that are missing and presumably destroyed. Professor Jon Yngve Hardeberg at The Norwegian Colour and Visual Computing Laboratory at GUC is a driving force in this research.
Hardeberg hopes that the reconstruction of lost artworks by enhancement of the quality of preserved photographs, can give us new insights into and knowledge of art history.
"It will not be an exact reproduction of the colour, texture and details of the original painting, but the painting’s history will still be told in a better and more detailed way than a poor quality photograph does," he explains.
Super-resolution and colour correction
This project is the first attempt to recreate lost art with such methods, and opens new possibilities regarding the use of colour imaging technology within the art world.
The method is a combination of super-resolution and colour correction. With the help of mathematical algorithms, a colour corrected high quality image is created, where the resolution is much higher than in the original photo. To manage this, the researchers collect information about texture, details and colours from high resolution and colour corrected digital images of comparable paintings.
"We use both low resolution and high resolution fragments of images to train the algorithms," Hardeberg says.
The low resolution images are scanned from older art books, while the high resolution images stems from modern digital colour corrected images of the same paintings. The statistical relationship between these data sets are then used for reconstructing similar paintings.
Peter Paul Rubens
The goal is for the image to better match the original painting both in structure and in colour.
"In the experiments, we used several images of Peter Paul Rubens. These were scanned from art books from the interwar period and then treated with the various algorithms," says Hardeberg. Such images are naturally of varying quality, but the results are very promising.
According to the researchers, this method opens new fields of use for colour imaging. The next step in the process will be printing the new, high-resolution images. If the results are satisfying, it may be possible to exhibit reproductions of lost works of art next to real paintings.
"Maybe one day we can contribute to an art exhibition where a recreated Harlequin Head can be admired along with other of Picasso's later work," Hardeberg says.
------------
Read the Norwegian version of this article at forskning.no
Scientific links
- Computer-Aided Reclamation of Lost Art, A. Fusiello et al. (Eds.): Computer Vision - ECCV 2012 Workshops and Demonstrations, Part I, Lecture Notes in Computer Science, Volume 7583, pp. 551–560 (Abstract)
- Learning super-resolution from misaligned examples. Colour and Visual Computing Symposium (CVCS), IEEE, pp 1-5, 2013. (Abstract)