THIS ARTICLE/PRESS RELEASE IS PAID FOR AND PRESENTED BY the Norwegian centre for E-health research - read more
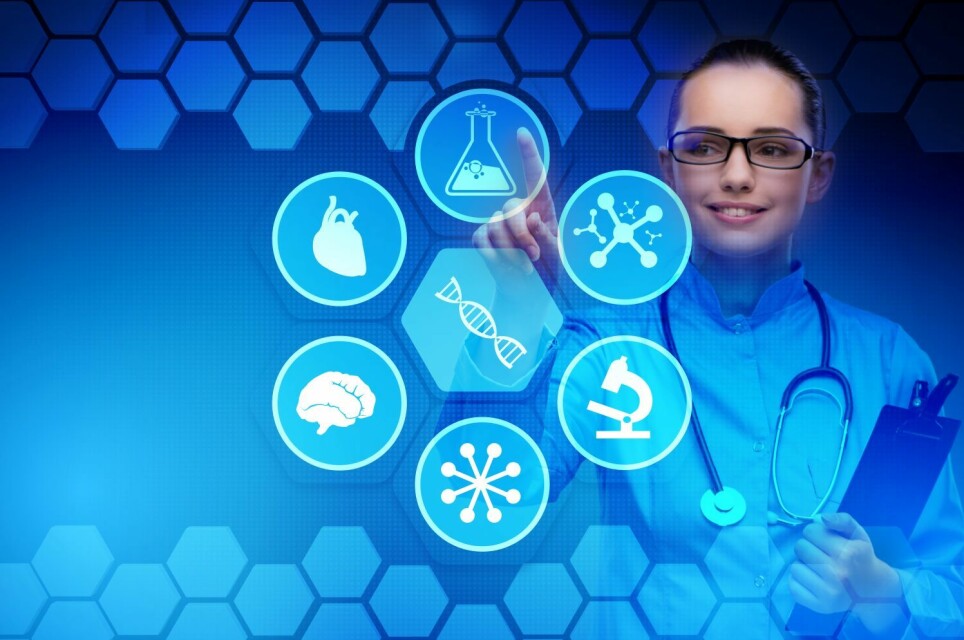
More thorough analyses of health data can boost patient care
Algorithms that analyse health conditions can be a new and better method of tailoring treatment.
Most data about the patient are unstructured. This applies to doctor's notes, radiology and pathology reports, discharge letters and medical images that doctors and other health personnel store in our electronic health record.
“We estimate that 80 percent of patient data is unstructured. This presents some challenges,” says Alexandra Makhlysheva.
Together with other researchers at the Norwegian Centre for E-health Research, she has written a report on electronic phenotyping in patient treatment.
Phenotype is in genetics the characteristics of an individual that can be observed directly, such as appearance, colour, size, intelligence and state of health. With the help of systematic analyses, researchers can thus find specific properties of health conditions from health data.
Electronic phenotyping algorithms are computer programs that analyse patient data. The goal is to find patterns and characteristics of a condition in patients or predict the risk of the condition.
Automatic analysis
Patients with the same disease may respond differently to a treatment. To give the best targeted treatment to each individual, researchers need large sets of data.
The researchers study data in the electronic health record as well as other sources, to get the most information about patients.
“We can get this information, for example, from lab samples, medicines, notes and genomic data,” says project manager Alexandra Makhlysheva.
“Using machine learning analysis, we find characteristics shared by persons with similar conditions. Then we can uncover how a treatment works or prevent diseases,” the researcher explains.
In analyses of text and language, they use computer programs with natural language processing.
What is best, for whom?
The researchers studied elderly patients with several chronic diseases. Some were referred to a patient-centred health care team, in a project led by Professor Gro Berntsen at the Norwegian Centre for E-health Research.
An algorithm was developed, which collected and analysed a large data set from health care teams, hospitals and municipal health services. This algorithm found out the benefits the patients had from the treatment. Based on that, the researchers found the criteria for referring new patients to the intervention.
Improving administrative operations
Findings from electronic phenotyping algorithms can be useful for health professionals in the primary and specialist health services, as well as for public health agencies at the national level.
But, digging for data can also be used for administrative purposes.
“In a hospital, we can save time for clinicians and patients, save money and improve the workflows. An algorithm can analyse how a patient is treated, in what order things are done and find out which treatment is best. For example, a person with kidney failure will have a specific patient flow at the clinic. With phenotyping, all procedures and outcomes can be analysed. We can also see how much money is spent on each step.”
“Will the machines first control us, and then take over the control of treatment?”
“No, the machines can help provide support for decisions. It is the clinician or the administrative staff who must decide what they want to do - or not do,” says Makhlysheva.
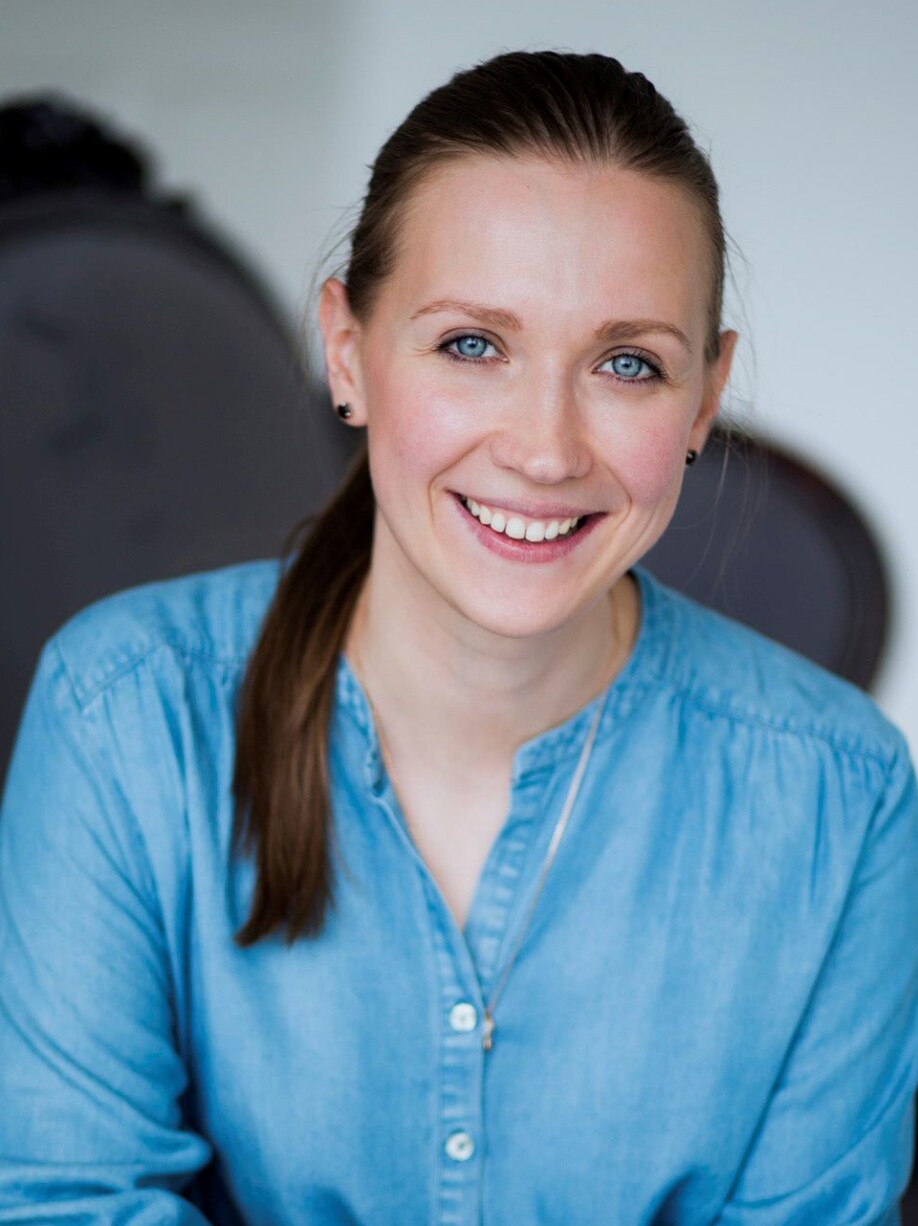
Clinical coding support
She points to clinical coding support as another area where algorithms can help.
After each patient contact, the doctor registers diagnostic codes that describe treatments and procedures.
“These codes are collected and used at a higher level to measure activity in the hospitals, as well as determine the hospitals' reimbursement from the state. But, about 40 percent of coding might be wrong,” she says.
Incorrect coding makes it difficult to direct resources in the health care system in an efficient way. A phenotyping algorithm can analyse the patient’s health record and come up with suggestions for diagnostic codes that help the clinician make the right choice.
“An algorithm can read through lots of patient records and never gets tired. It will simplify the work of code controllers,” she says.
Makhlysheva says that they have received funding for the project ClinCode - Computer-assisted clinical ICD-10 coding for improving efficiency and quality in healthcare. They will create an algorithm for ICD-coding support and collaborate with a health record vendor to find out how it can be built into the record.
“Research in this field is evolving. We need access to more health data. It is also necessary to protect privacy and to get legislation in place to use phenotyping in clinical practice in Norway,” she concludes.
Reference:
Alexandra Makhlysheva et.al.: Exploring electronic phenotyping for clinical practice in Norwegian settings. Report from The Norwegian Centre for E-Health Research, 2020.
———
Read the Norwegian version of this article at forskning.no
See more content from the Norwegian centre for E-health research:
-
Technology in the health and care sector: "This is not just about new gadgets"
-
Five Nordic and Baltic countries take a major step toward the future of health research
-
A digital chatbot can help you stay fit
-
Researchers' advice for better healthcare services: Listen to the patient!
-
Half of those who received mental health care found errors in their medical records
-
AI can understand your medical records: A new language model could revolutionise healthcare