THIS CONTENT IS BROUGHT TO YOU BY the Norwegian centre for E-health research - read more
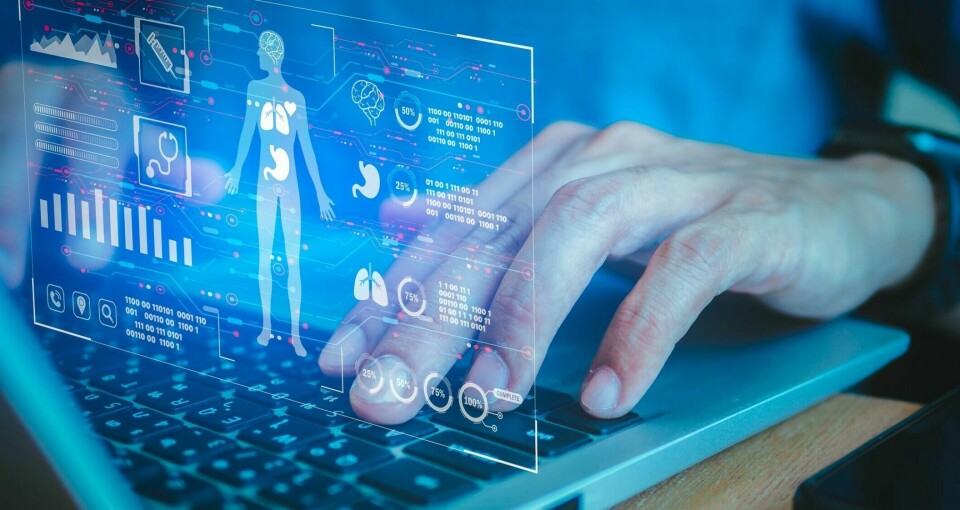
When artificial intelligence guards privacy, it may be the path to improved healthcare
How to research health data without spreading health information you would prefer to keep to yourself? Technologies that promote privacy ensure researchers can learn from the data while privacy is maintained.
Insight into health data can change the game rules for research and patient care. But the path there is not without obstacles.
We aim to utilise health data to provide better treatment and use resources more eficiently. However, this often clashes with strict regulations for using health information. Fortunately, there are technologies that take care of this. We call them privacy-enhancing technologies. They open the door for secure and efficient analysis of health data.
A new research report from the Norwegian Centre for E-health Research presents two such technologies: federated learning and synthetic data.
The report explores how these two technologies can solve the problem of accessing high-quality health data. At the same time, there must be enough data for research and service development.
Health Data: A strictly guarded goldmine
The healthcare service produces vast amounts of data. If used correctly, this information can aid research. Moreover, it can improve diagnostics and ensure resources in the healthcare service are smartly utilised.
Artificial intelligence (AI) has proven to be a powerful tool for analysing large amounts of health data quickly and efficiently. This is especially true for machine learning.
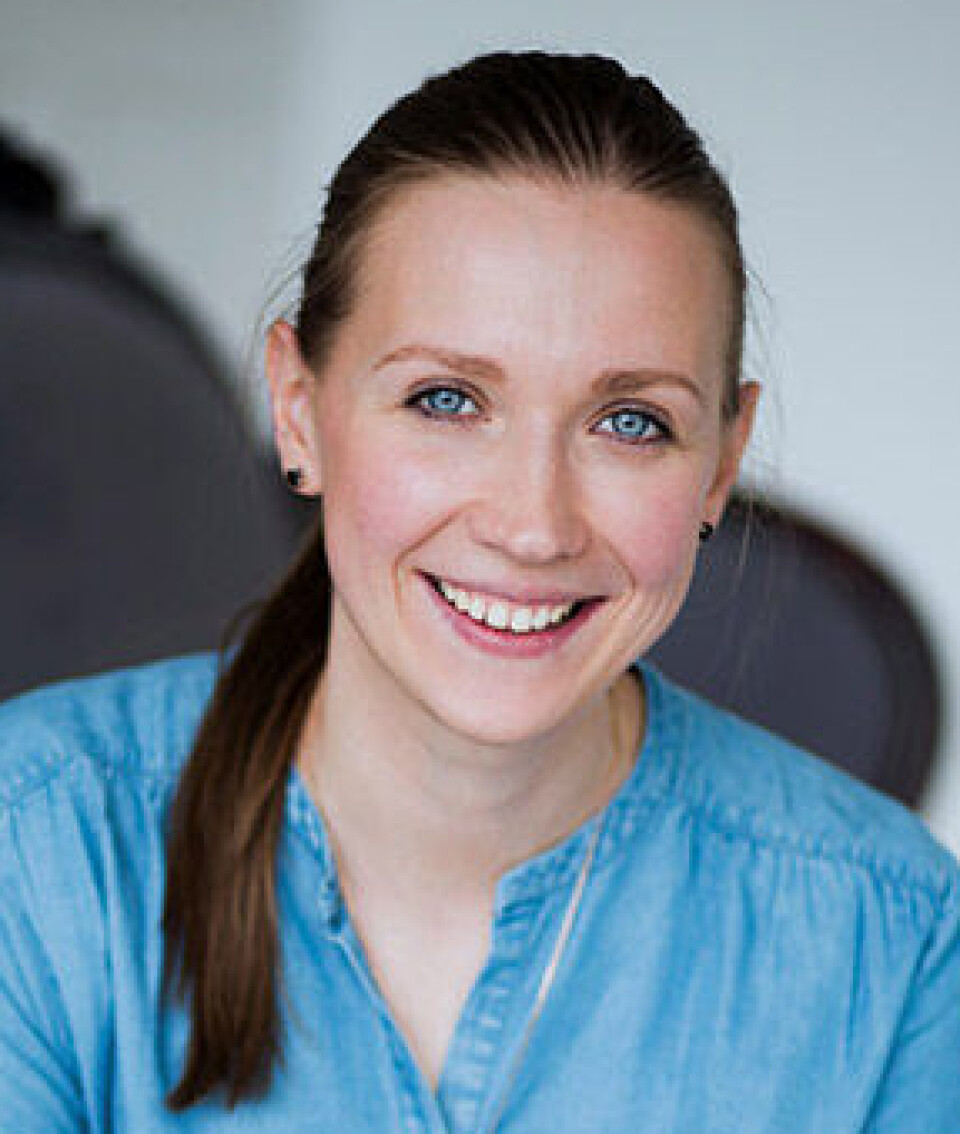
Health information is not just valuable – it's also sensitive. Its use is strictly regulated. Accessing health data for secondary use is difficult.
“Ensuring compliance with privacy and data security regulations requires a significant amount of time and effort,” says Alexandra Makhlysheva, senior adviser at the Centre's Health Data and Analysis Department. She is one of the authors of the report.
Privacy and data security make it harder to access data for training and to spread the use of AI in health and care services.
“Privacy-enhancing technologies can help collect, process, analyse, and share data while maintaining data security and privacy,” Makhlysheva says.
Collaboration without data leakage
Federated learning is a type of machine learning that allows us to analyse data where it is already stored, preventing the data from being seen or shared with others.
This technology provides better control over one's data, enhanced privacy, and the opportunity to analyse larger and more representative datasets. This can assist in making better treatment decisions. It can provide better healthcare to patients, regardless of where they are treated or what they suffer from.
Using federated learning also makes it easier to meet the requirements for how data must be treated to comply with privacy principles.
However, the technology comes with some issues. For instance, there are differences in data formats and ICT infrastructures in the organisations collaborating on data analysis. The learning also puts increased pressure on communication systems and can pose risks to data security.
“Ensuring data security across all systems is challenging. Therefore, it's important to have a secure communication system. There are also several mechanisms that can be used to strengthen data security and privacy in federated systems,” Makhlysheva says.
Realistic but anonymous alternatives
Synthetic data are artificially generated data produced by training a generative machine learning model with real data. The model being generative means it uses its learning to create new content.
These data retain the statistical properties of the original dataset but do not contain information about actual patients.
This is useful when we lack sufficient real training data. It provides larger and more representative data foundations while reducing the risk of identifying the individuals behind the data. Combined with other privacy-enhancing methods, synthetic data can lower the risk of privacy breaches.
Synthetic data can be used in health and care services to develop machine learning models. Later, researchers can check how the models perform against real data.
Sharing such data openly is also beneficial, allowing more people to use them for research. However, using synthetic data is not without problems. It can introduce new biases. Researchers must ensure statistical similarity with original data and consider cost-effectiveness.
“How useful it is to use synthetic data in health and care services varies by situation. We need to thoroughly test them to ensure they fit our needs while keeping the risk of privacy breaches low,” Makhlysheva says.
Need better tools
“Federated learning and synthetic data are excellent tools for protecting privacy when analysing health data. For these technologies to be used in practice, we need more research,” Makhlysheva says.
She emphasises the need for improving tools and methods, as well as testing them in practice, to truly unlock their full potential and assist in utilising AI safely and effectively in healthcare.
“The technologies should improve health and care services for everyone – individuals, the service, and society at large – but they must continuously evolve,” she says.
Reference:
Makhlysheva et al. Personvernfremmende teknologier for bruk av kunstig intelligens i helse- og omsorgstjenesten (Privacy-enhancing technologies for the use of artificial intelligence in health and care services), NSE-report 2023-04, 2023.
———
Read the Norwegian version of this article on forskning.no
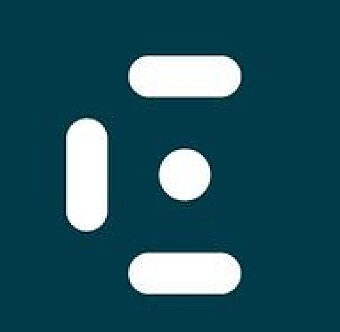
This content is paid for and presented by the Norwegian centre for E-health research
This content is created by the Norwegian centre for E-health research's communication staff, who use this platform to communicate science and share results from research with the public. The Norwegian centre for E-health researchis one of more than 80 owners of ScienceNorway.no. Read more here.
More content from the Norwegian centre for E-health research:
-
Half of those who received mental health care found errors in their medical records
-
AI can understand your medical records: A new language model could revolutionise healthcare
-
People are receiving more digital healthcare in Europe
-
How to find motivation to exercise
-
Health data is difficult for users to access
-
The teams that help people with mental health problems struggle with digital issues